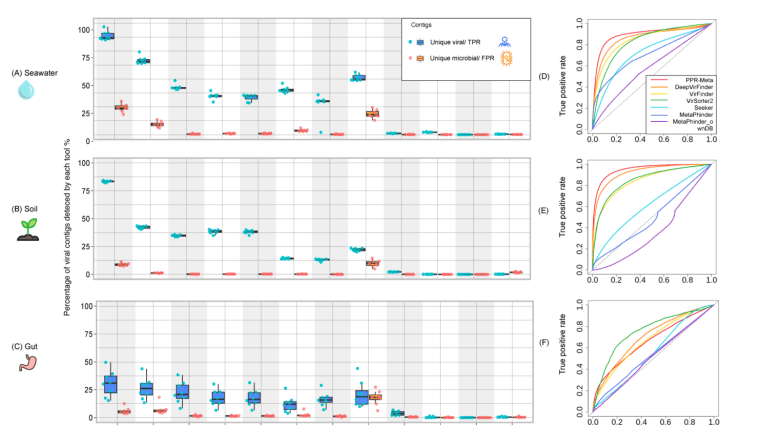
- Light
- Life
- Liberty
- Publications
- Research
Published: | By: Marcel Baecker and Ling-Yi Wu
A novel way to compare virus hunting tools
Viruses of microbes (VoMs) are the most abundant and diverse living entities on Earth, with significant potential for various biological applications such as antimicrobial drugs, biotechnology, and bioremediation. However, identifying VoMs is a complex task akin to finding a needle in a haystack. Numerous bioinformatic tools have been developed to address this challenge, each utilizing different methods and signals, which complicates the selection of a good tool for biologists.
Benchmarking overview
Image: Wu, LY., Wijesekara, Y., Piedade, G.J. et al.The challenge is to find a bioinformatic tool that detects many viral sequences (high sensitivity) while not detecting false positives, i.e., sequences from cellular organisms (specificity). Previous benchmarking studies used testing datasets based on sequences from known viruses, e.g. from RefSeq, yet this leads to overlap between testing and training/reference datasets since most tools are developed based on known viruses. However, the complexity and diversity of naturally occurring viruses is much higher than that of known viruses. To overcome these limitations, we introduced a novel evaluation method using paired real-world virome and total metagenome datasets from the same environment. In this approach, the viral particle-enriched datasets serve as ground truth positives, while the total metagenomic datasets represent ground truth negatives. We removed overlapping sequences from both viral and microbial datasets, as they could represent cellular sequences in the viral fraction that escaped DNase treatment or viral sequences in the cellular fraction (prophages, active infections). This method provides a new perspective of assessment of the tools' performance, guiding researchers in choosing the most appropriate tools for their specific needs.
The quality of our ground truth datasets relies on the purity of the selected metagenomic data. We selected studies with robust procedures to separate viruses from microbes, including physical size fractionation through 0.22 μm filters and DNase treatment of the virome to remove free DNA. Finding these datasets was not easy. In the end, we used a soil datasetExternal link with adequate procedures for virus-microbe separation and an Antarctic marine datasetExternal link from an understudied region in collaboration with Gonçalo Piedade and Corina Brussaard from NIOZ.External link
Our study revealed that all tools detect high-quality viral sequences more easily than short fragments and that machine-learning tools were much better than homology-based tools. Thus, we are currently developing a new CNN tool, Jaeger, to hunt those viruses with even higher precision. For updates, visit https://github.com/Yasas1994/JaegerExternal link. Stay tuned!
Two affiliated PhD researchers led this study:
Image: Ling-Yi Wu
Ling-Yi Wu is a PhD researcher at the MGX group at the Utrecht University, Netherlands. She is interested in hunting viruses in the wild. After finding a new virus, she likes to investigate their lifestyles and their influences on higher organisms across diverse environments, including marine seawater, soil, host-associated systems, and extreme environments. Utrecht University funds her work in the context of the Netherlands Center of One HealthExternal link.
Image: Yasas Wijesekara
Yasas Wijesekara is a PhD researcher at the University of Greifswald. He shares Lingyi's interest in virus hunting. He also likes to explore viral lifestyles and functions across different environments, especially in host-associated systems. He is passionate about applying machine learning tools to solving life science puzzles. The European training network VIROINFExternal link funds his project.
Original Publication
Ling-Yi Wu, Yasas Wijesekara et al. Benchmarking bioinformatic virus identification tools using real-world metagenomic data across biomes. Genome Biology 25, 97 (2024). https://genomebiology.biomedcentral.com/articles/10.1186/s13059-024-03236-4External link